Deep Learning Theories for Problems with Low–Dimensional Structures
Topic | Learning theory Low-dim structure |
---|---|
Format | Hybird |
Location | DSDSNUSS16 07-107 |
Speaker | Liu Hao (HKBU) |
Time (GMT+8) |
Abstract
Deep neural networks have demonstrated a great success on many applications, especially on problems with high-dimensional data sets. In spite of that, most existing theories are cursed by data dimension and cannot explain such a success. To bridge the gap between theories and practice, we exploit the low-dimensional structures of data set and establish theoretical guarantees with a fast rate that is only cursed by the intrinsic dimension of the data set. This presentation addresses our recent work on function approximation and operator learning by deep neural networks. The first part function approximation on low-dimensional manifolds. For Sobolev functions defined on a low-dimensional manifold, we show that neural networks can approximate both the function value and its gradient well. The network size critically depends on the intrinsic dimension of the manifold and only weakly depends on the ambient dimension. In the second part, we consider a general encoder-decoder framework to learn Lipschitz operators between infinite dimensional spaces by feedforward neural networks. Such a framework covers most scenarios in real applications. We develop non-asymptotic upper bounds for the generalization error of the empirical risk minimizer. When the problem have low-dimensional structures, our error bounds have a fast rate depending on the intrinsic dimension. Our results show that neural networks are adaptive to the low-dimensional structures of the problem.
Reference
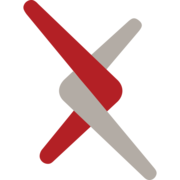
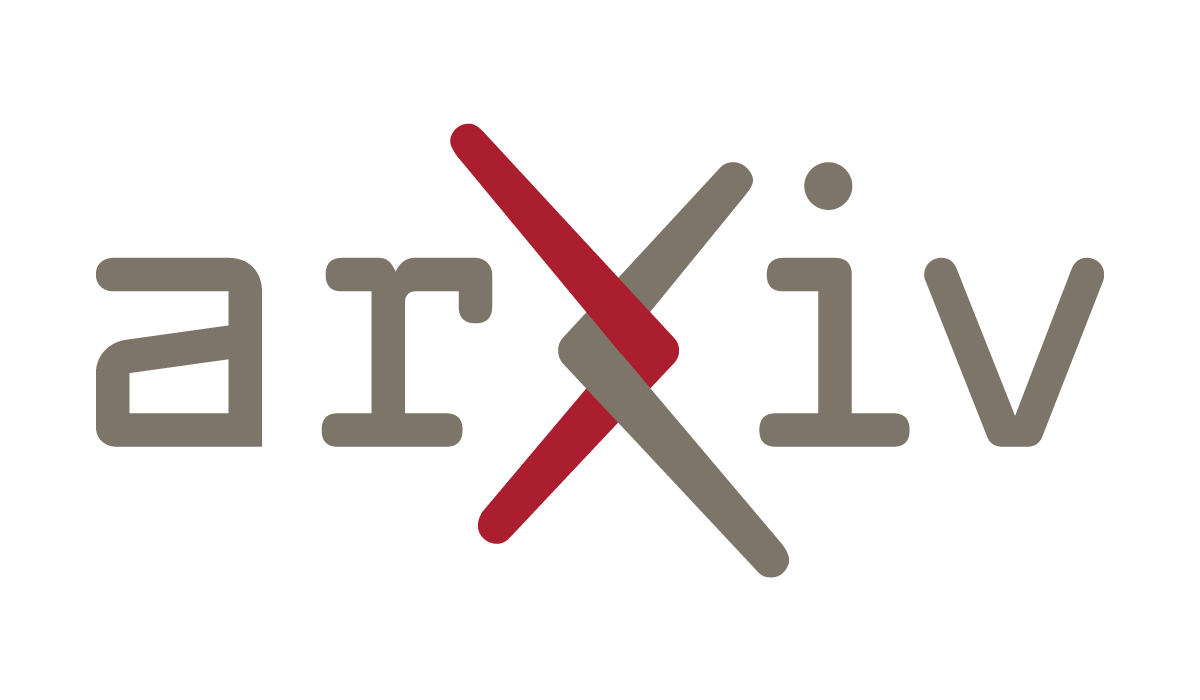
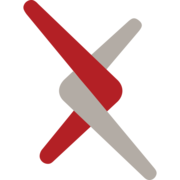
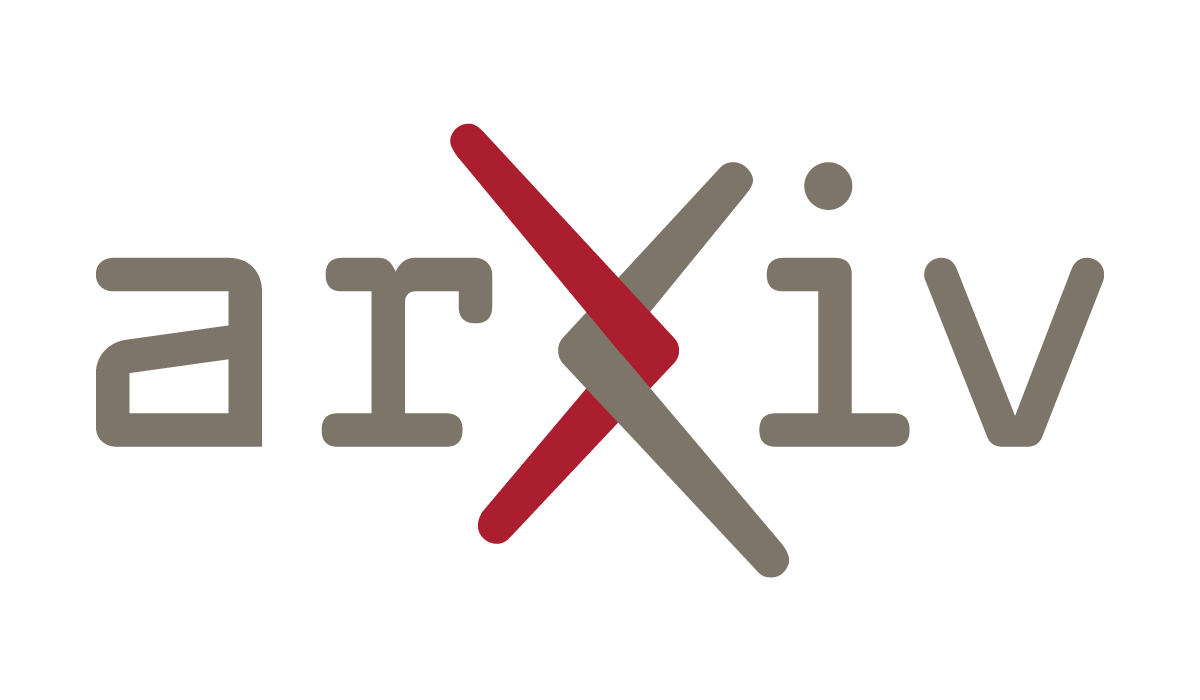