Robust AI-aided Imaging Models with Unpaired Data
Topic | CryoEM |
---|---|
Format | Hybird |
Location | DSDSNUSS16 07-107 |
Speaker | Bao Chenglong (THU) |
Time (GMT+8) |
Abstract
The observations in practical imaging systems always contain complex noise such that classical approaches are difficult to obtain satisfactory results. In recent years, deep neural networks directly learned a map between the noisy and clean images based on the training on paired data. Despite its promising results in various tasks, collecting the training data is difficult and time-consuming in practice. In this talk, in the unpaired data regime, we will discuss our recent progress for building AI-aided robust models and their applications in image processing. Leveraging the Bayesian inference framework, our model combines classical mathematical modelling and deep neural networks to improve interpretability. Experimental results on various real datasets validate the advantages of the proposed methods. Finally, I will report the recent progresses on solving the preferred orientation problems in cyroEM using the developed tools.
Reference:
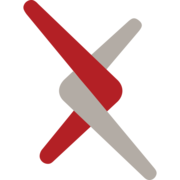
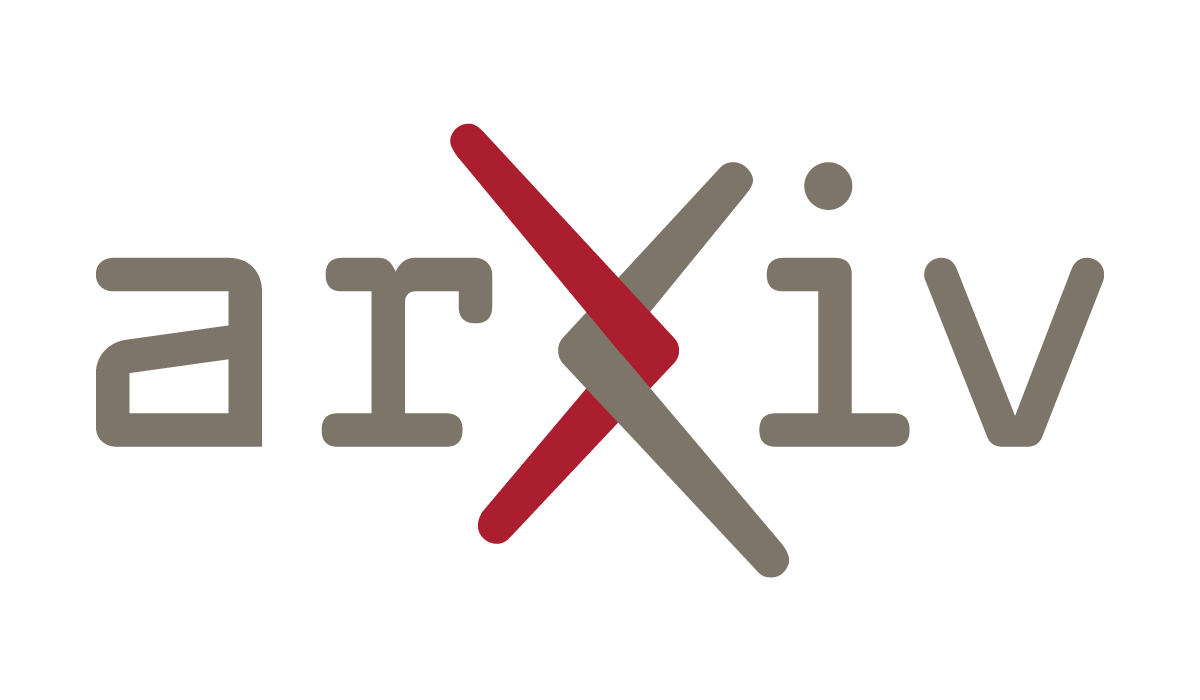
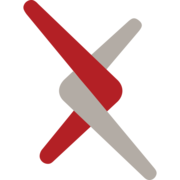
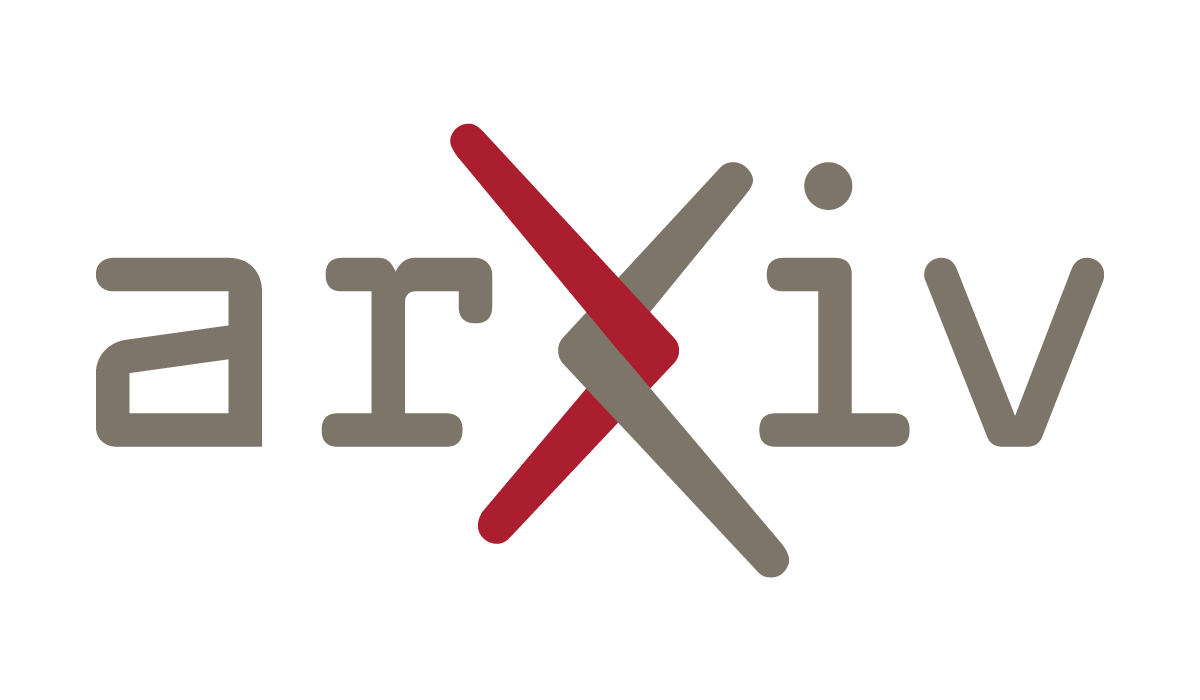
Procedure of cyroEM:
One mentioned data set:
